Data Demystified, Part 1: Getting started with data analytics & business Intelligence
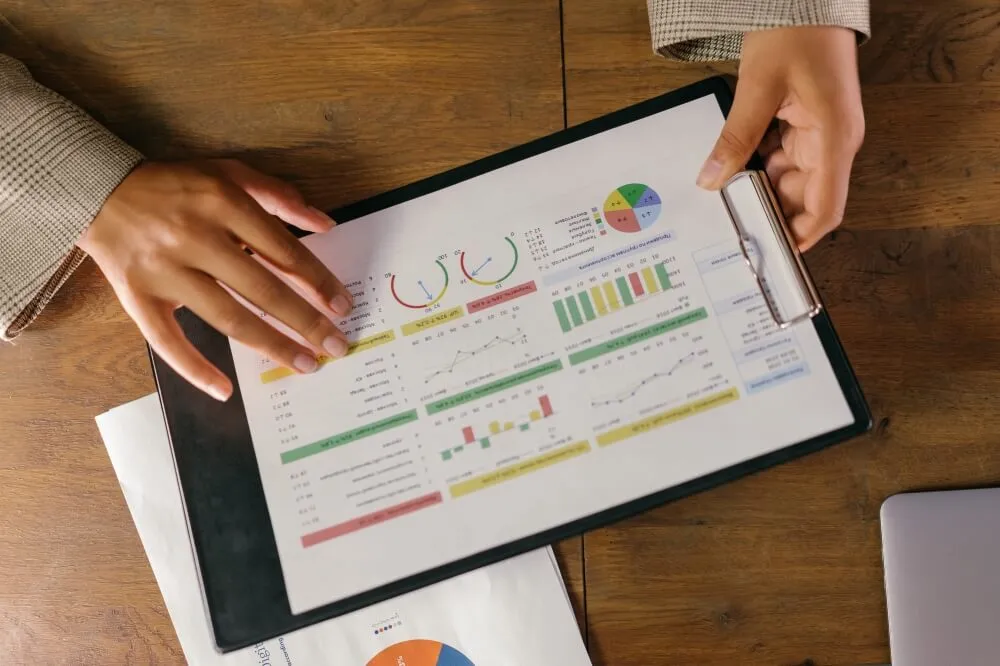
The way organizations run their data analytics and business intelligence (BI) functions is changing.
Nowadays, it’s not uncommon to see Heads of Finance, Chief Operating Officers, Chiefs of Staff, Directors of Product, and other non-technical business leaders overseeing data analytics and BI. It’s an arrangement that’s becoming especially common in young, high-growth companies that have a strong need for data investments.
This is a big contrast from past norms, where decisions about BI were most often left to engineering teams. It’s an exciting change, but this shift also (understandably) makes some non-technical leaders a bit nervous. The risks and rewards are high, for both you and the business, and there’s a lot that can go wrong.
But if you get it right, it will be a catalyst for massive career growth and development. 🚀
With that in mind, we’re going to demystify the process of running data analytics and business intelligence. We’ll start with the “Why?” behind the shift toward non-technical business leaders running BI and data analytics, and finish up with the core responsibilities you’ll be expected to take on.
Why would a non-technical leader be asked to run data analytics and BI?
Before you can lay the foundational strategy for data analytics and business intelligence or make decisions about hiring and technology, it’s helpful to think about how you got here.
These are the most common scenarios that we see, but note that they’re not mutually exclusive – your company could fit into one or more of these categories:
- An early stage company is going for their Series A (or even B) funding. For that to happen, the organization needs accurate, reliable operational performance data. For a deal to get done, both the company founders and their prospective investors need direct line of sight into company key performance indicators (KPIs).
- An early stage company just received their Series A funding round. External reporting is now required for the first time ever. The Board requires updates every quarter and the data needs to be accurate and reflective of company performance… or heads may roll.
- The organization’s former data leader has left the company. This person probably held all the “secrets” about how everything works behind the scenes, but someone else needs to take the reins ASAP.
- The data function needs some TLC in general. Perhaps it wasn’t managed well, so teams are working in disconnected silos that lead to contradictory reporting. This causes people to distrust the data and question its accuracy. From there, people might even say, “Screw it,” and make business decisions based on instincts and hunches. 😱
In any of the above situations, it makes sense that someone with the right business acumen and semi-technical skillset could be called on to take over data analytics and business intelligence. If you've recently been tasked with data-related responsibilities, you're probably a leader who’s comfortable working across departments and navigating complex situations.
Keep this in mind, especially if you feel stuck or frustrated.
Your responsibilities when building a business intelligence & data analytics function
So, you’re ready to jump in and “own” business intelligence and data throughout your organization. Here’s a quick primer on some of the most important responsibilities that you have on your plate:
1. Develop cross-functional partnerships
A core part of your job will be working with different functions within the organization to help them establish solid data practices. Effective data analytics and BI practices can’t happen in a silo, so you’ll need to work cross-functionally to establish good data practices among the company’s various departments.
The choices you make around data analytics and BI will have a big impact on multiple parties within your organization, so it’s vital that the people who work in those functions have a say in the process. Determine which functions need to be engaged (like sales, marketing, product, engineering, and finance.) Then, make a commitment to fostering those partnerships.
The result will be increased trust in company-wide data so that users don’t have to worry about accuracy.
2. Be a champion for data hygiene
Data hygiene practices ensure that your company has clean data that’s consistent, accurate, and error-free (at least to a reasonable degree.)
Here are two common examples of good data hygiene practices that you should strive for:
Record-level hygiene, aka adherence to operational processes and policies that impact downstream data assets. For example, good Salesforce data hygiene practices amongst SDRs and sales reps (e.g., keeping leads and opportunities up to date) will help sales leaders manage their teams more effectively.
Here’s another example: If you’re using a customer support tool like Zendesk, service representatives need to close out their tickets on time so that resolve times are accurately captured, and ensure tickets are tagged with the right triage level to ensure SLA adherence is calculated accurately.
Your cross-functional partnerships will be helpful for this, because you’ll need to work with different functional leads to ensure they enforce best practices and educate their teams on why data hygiene is important.
Overall cross-functional agreement on key metric definitions so that different teams don’t end up “reading from different sheets of music” during the same performance, so to speak.
All of these expectations need to be developed alongside functional leaders and communicated clearly to employees, which brings us to the next responsibility you’ll have.
3. Implement clear communication and change management standards
Here’s a (very) short list of some of the things you’ll want clear communication around:
- How stakeholders’ needs will be prioritized
- How data requests should be made
- Expectations for data requests and delivery timelines
- How to handle changes, e.g. a process change (or schema-related change) from an upstream team that has a downstream impact on business-critical reports
One hidden (but potentially powerful) benefit of good communication and change management is that it will buy you magnanimity and empathy when things hit a bump in the road. Your cross-functional partners are ultimately, for better or for worse, going to judge your performance leading data. Good communication, and the relationships that develop as a result of it, can afford you a bit of slack.
4. Hire the right team for the right job
The definition of “right team” as it relates to hiring and organizational structure will depend on your company’s needs and budget.
Regardless, you’ll be responsible for sourcing and managing the people who will be responsible for deploying your data analytics and business intelligence strategy. We recommend that you start small in the beginning – one or two people, tops. You can also work with an outside consulting/services firm on an as-needed basis.
This can be an effective, affordable way to make sure the data function runs smoothly, though we’d recommend against outsourcing for too long. Be sure to seek references and carefully vet any outside firm as thoroughly as you would a new hire, especially their technical and communication skills.
Job descriptions and roles in data analytics and BI can be notoriously vague; there’s a lot of disagreement about what different positions do. For a deeper dive into the different types of roles you’ll want to consider when building out your data team and how to build your team efficiently for maximum impact, check out this resource.
5. Determine the best data deployment model
Be sure to spend some time thinking critically about this one, because how you approach deployment sets the stage for the entire data analytics and business intelligence function:
👤 Will you aim for a self-service strategy that, if set up and supported properly, requires fewer people to operate?
👥 Or will you opt for a more traditional environment that’s tightly controlled and requires a larger team of dedicated data gurus to run?
Note that there’s also shades of gray within both of those questions – they aren’t mutually exclusive.
6. Develop onboarding and training programs
Don’t skimp on training – a carefully thought out approach will make onboarding more efficient, simplify requests, and take pressure off the data team. Training is especially crucial if you choose a self-service approach to data and BI.
This includes:
- Proactively thinking about what types of questions end-users, both technical and non-technical alike, might have
- Developing user-friendly guides for end-users, including documentation that shows them how to access and work with data assets
- Incorporating sessions on how to locate and work with data during employee onboarding
You should also curate an environment where asking questions is strongly encouraged. There’s a lot to learn about data analytics and BI. Educating your colleagues will go a long way toward developing a company culture where people feel capable and empowered when it comes to working with data.
7. Embrace that your data journey will evolve over time
Building great data analytics and business intelligence isn’t a one-and-done deal. You won’t get it 100% right from the start, and there are many reasons for that:
- The initial data models you build definitely won’t be able to answer the entire universe of questions that exist within the company - there are an infinite number of problems that can arise which data can be used to help clarify and solve.
- If your business pivots or expands into new lines of business, your schema could shift considerably, and extensive work will need to be done to ensure that data is normalized in reporting.
- Realistically, you’ll need to prioritize investments in high-quality, high-confidence data models and reporting. It’s not uncommon for initial data initiatives to focus on the top 10% - 30% of opportunities. Your job as a data leader is to help the organization prioritize and act upon the most valuable of these.
Basically, mistakes will be made, you’ll learn a ton, and you’ll adjust as needed.
If you lean in and learn how to “do” data analytics and BI correctly, both you and the company will benefit greatly. Remember what we said at the beginning: non-technical business leaders who can step up to the plate and run a data analytics and business intelligence function are poised to become indispensable members of their teams.
This is the first article in our Data Demystified series, where you’ll get a clear roadmap to follow for your early days as a data leader. Stay tuned for the next installment, where we’ll explain How to Set Your Data Strategy, including the exact questions you should ask to set a strong foundation.
Scale your most ambitious SEO strategies
Use AI-powered workflows to turn your boldest content strategies into remarkable growth
Get the latest on AI content & marketing
Get the latest in growth and AI workflows delivered to your inbox each week